- Crunchy PostgreSQL Operator DocumentationAvailable FormatsNavigation
- Installation
- Ansible
- PostgreSQL Operator Monitoring
- Other Methods
- Upgrade
- Release Notes
- Postico 1 3 3 – A Modern Postgresql Client Documentation Download
- Postico 1 3 3 – A Modern Postgresql Client Documentation Requirements
- Postico 1 3 3 – A Modern Postgresql Client Documentation Example
PostgreSQL 8.1.23 Documentation; Prev: Fast Backward: Fast Forward: Next: II. PostgreSQL Client Applications. This part contains reference information for PostgreSQL client applications and utilities. Not all of these commands are of general utility, some may require special privileges. The common feature of these applications is that they can.
Run your own production-grade PostgreSQL-as-a-Service on Kubernetes!
Latest Release: 4.5.0 https://dantuisetdazz1982.wixsite.com/gaysoftware/post/adobe-image-reader-software-free-download.
- Postico is a modern database app for your Mac. Postico is the perfect tool for data entry, analytics, and application development. – connect to Postgres.app.
- 1 Unconference schedule by Time and Room; 2 Notes/Minutes. 2.1 Data Loading for Data Warehousing. 2.1.1 Features NOT implemented yet; 2.1.2 pgbulkload; 2.1.3 Ideas; 2.1.4 Idea that Slony team had; 2.1.5 Present Pain Points; 2.2 Which new illustrations do we need in documentation? 2.3 Schema Management. 2.3.1 Some approaches; 2.3.2 More modern.
- On the other hand, Postico is detailed as 'A modern PostgreSQL client for OS X'. Postico provides an easy to use interface, making Postgres more accessible for newcomers and specialists alike. Postico will look familiar to anyone who has used a Mac before. Just connect to a database and begin working with tables and views.
The Crunchy PostgreSQL Operator automates and simplifies deploying and managing open source PostgreSQL clusters on Kubernetes and other Kubernetes-enabled Platforms by providing the essential features you need to keep your PostgreSQL clusters up and running, including:
PostgreSQL Cluster Provisioning
Create, Scale, & Delete PostgreSQL clusters with ease, while fully customizing your Pods and PostgreSQL configuration!
High Availability
Safe, automated failover backed by a distributed consensus based high-availability solution. Uses Pod Anti-Affinity to help resiliency; you can configure how aggressive this can be! Failed primaries automatically heal, allowing for faster recovery time.
Support for standby PostgreSQL clusters that work both within an across multiple Kubernetes clusters.
Disaster Recovery
Backups and restores leverage the open source pgBackRest utility and includes support for full, incremental, and differential backups as well as efficient delta restores. Set how long you want your backups retained for. Works great with very large databases!
TLS
Secure communication between your applications and data servers by enabling TLS for your PostgreSQL servers, including the ability to enforce that all of your connections to use TLS.
Monitoring
Track the health of your PostgreSQL clustersusing the open source pgMonitorlibrary.
PostgreSQL User Management
Quickly add and remove users from your PostgreSQL clusters with powerful commands. Manage password expiration policies or use your preferred PostgreSQL authentication scheme.
Upgrade Management
Safely apply PostgreSQL updates with minimal availability impact to your PostgreSQL clusters.
Advanced Replication Support
Choose between asynchronous replication and synchronous replication for workloads that are sensitive to losing transactions.
Clone
Create new clusters from your existing clusters or backups with pgo create cluster --restore-from
.
Connection Pooling
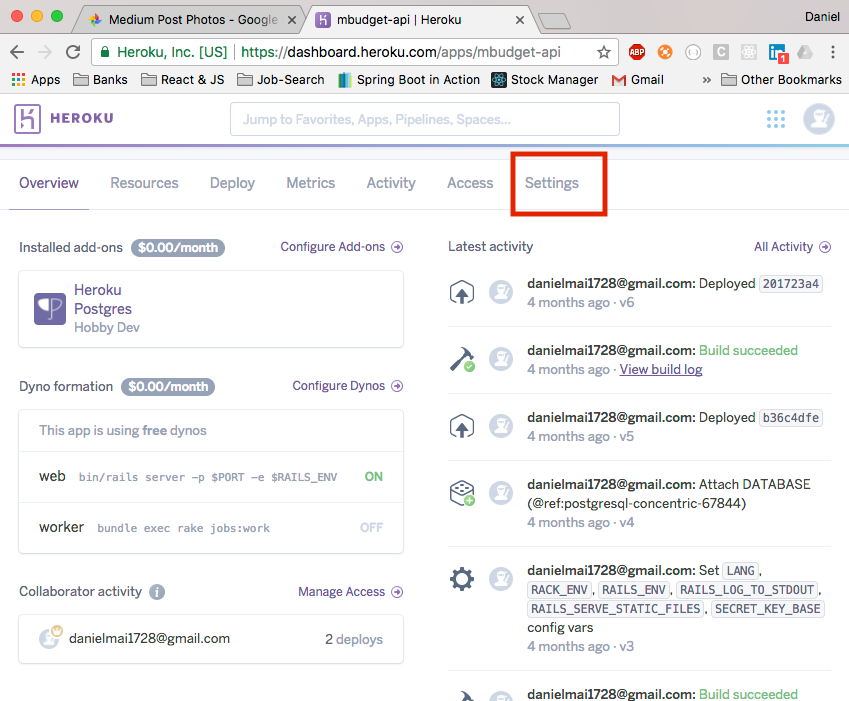
Use pgBouncer for connection pooling
Node Affinity
Have your PostgreSQL clusters deployed to Kubernetes Nodes of your preference
Scheduled Backups
Choose the type of backup (full, incremental, differential) and how frequently you want it to occur on each PostgreSQL cluster.
Backup to S3
Store your backups in Amazon S3 or any object storage system that supports the S3 protocol. The PostgreSQL Operator can backup, restore, and create new clusters from these backups.
Multi-Namespace Support
You can control how the PostgreSQL Operator leverages Kubernetes Namespaces with several different deployment models:
- Deploy the PostgreSQL Operator and all PostgreSQL clusters to the same namespace
- Deploy the PostgreSQL Operator to one namespaces, and all PostgreSQL clusters to a different namespace
- Deploy the PostgreSQL Operator to one namespace, and have your PostgreSQL clusters managed acrossed multiple namespaces
- Dynamically add and remove namespaces managed by the PostgreSQL Operator using the
pgo create namespace
andpgo delete namespace
commands
Full Customizability
The Crunchy PostgreSQL Operator makes it easy to get your own PostgreSQL-as-a-Service up and running on Kubernetes-enabled platforms, but we know that there are further customizations that you can make. As such, the Crunchy PostgreSQL Operator allows you to further customize your deployments, including:
- Selecting different storage classes for your primary, replica, and backup storage
- Select your own container resources class for each PostgreSQL cluster deployment; differentiate between resources applied for primary and replica clusters!
- Use your own container image repository, including support
imagePullSecrets
and private repositories - Bring your own trusted certificate authority (CA) for use with the Operator API server
- Override your PostgreSQL configuration for each cluster
The Crunchy PostgreSQL Operator extends Kubernetes to provide a higher-level abstraction for rapid creation and management of PostgreSQL clusters. The Crunchy PostgreSQL Operator leverages a Kubernetes concept referred to as 'Custom Resources' to create several custom resource definitions (CRDs) that allow for the management of PostgreSQL clusters.
PostgreSQL containers deployed with the PostgreSQL Operator include the following components:
- PostgreSQL
- LLVM (for JIT compilation)
In addition to the above, the geospatially enhanced PostgreSQL + PostGIS container adds the following components:
Fnaf final hours 2 download. PostgreSQL Operator Monitoring uses the following components:
Additional containers that are not directly integrated with the PostgreSQL Operator but can work alongside it include:
For more information about which versions of the PostgreSQL Operator include which components, please visit the compatibility section of the documentation.
The Crunchy PostgreSQL Operator is tested on the following Platforms:
- Kubernetes 1.13+
- OpenShift 3.11+
- Google Kubernetes Engine (GKE), including Anthos
- Amazon EKS
- VMware Enterprise PKS 1.3+
Storage
The Crunchy PostgreSQL Operator is tested with a variety of different types of Kubernetes storage and Storage Classes, including:
- Rook
- StorageOS
- Google Compute Engine persistent volumes
- NFS
- HostPath
and more. We have had reports of people using the PostgreSQL Operator with other Storage Classes as well.
We know there are a variety of different types of Storage Classes available for Kubernetes and we do our best to test each one, but due to the breadth of this area we are unable to verify PostgreSQL Operator functionality in each one. With that said, the PostgreSQL Operator is designed to be storage class agnostic and has been demonstrated to work with additional Storage Classes. Storage is a rapidly evolving field in Kubernetes and we will continue to adapt the PostgreSQL Operator to modern Kubernetes storage standards.
One might need to insert a large amount of data when first populating a database. This section contains some suggestions on how to make this process as efficient as possible.
14.4.1. Disable Autocommit
When using multiple INSERTs, turn off autocommit and just do one commit at the end. (In plain SQL, this means issuing BEGIN at the start and COMMIT at the end. Armed forces laser sight module manual. Some client libraries might do this behind your back, in which case you need to make sure the library does it when you want it done.) If you allow each insertion to be committed separately, PostgreSQL is doing a lot of work for each row that is added. An additional benefit of doing all insertions in one transaction is that if the insertion of one row were to fail then the insertion of all rows inserted up to that point would be rolled back, so you won't be stuck with partially loaded data.
14.4.2. Use COPY
Use COPY to load all the rows in one command, instead of using a series of INSERT Install office di mac. commands. The COPY command is optimized for loading large numbers of rows; it is less flexible than INSERT, but incurs significantly less overhead for large data loads. Since COPY is a single command, there is no need to disable autocommit if you use this method to populate a table.
If you cannot use COPY, it might help to use PREPARE to create a prepared INSERT statement, and then use EXECUTE as many times as required. This avoids some of the overhead of repeatedly parsing and planning INSERT. Different interfaces provide this facility in different ways; look for 'prepared statements' in the interface documentation.
Note that loading a large number of rows using COPY is almost always faster than using INSERT, even if PREPARE is used and multiple insertions are batched into a single transaction. Drive scope 1 2 8 inch.
COPY is fastest when used within the same transaction as an earlier CREATE TABLE or TRUNCATE command. In such cases no WAL needs to be written, because in case of an error, the files containing the newly loaded data will be removed anyway. However, this consideration only applies when wal_level is minimal as all commands must write WAL otherwise.
14.4.3. Remove Indexes
If you are loading a freshly created table, the fastest method is to create the table, bulk load the table's data using COPY, then create any indexes needed for the table. Creating an index on pre-existing data is quicker than updating it incrementally as each row is loaded.
If you are adding large amounts of data to an existing table, it might be a win to drop the indexes, load the table, and then recreate the indexes. Of course, the database performance for other users might suffer during the time the indexes are missing. One should also think twice before dropping a unique index, since the error checking afforded by the unique constraint will be lost while the index is missing.
14.4.4. Remove Foreign Key Constraints
Just as with indexes, a foreign key constraint can be checked 'in bulk' more efficiently than row-by-row. So it might be useful to drop foreign key constraints, load data, and re-create the constraints. Again, there is a trade-off between data load speed and loss of error checking while the constraint is missing.
What's more, when you load data into a table with existing foreign key constraints, each new row requires an entry in the server's list of pending trigger events (since it is the firing of a trigger that checks the row's foreign key constraint). Loading many millions of rows can cause the trigger event queue to overflow available memory, leading to intolerable swapping or even outright failure of the command. Therefore it may be necessary, not just desirable, to drop and re-apply foreign keys when loading large amounts of data. If temporarily removing the constraint isn't acceptable, the only other recourse may be to split up the load operation into smaller transactions.
14.4.5. Increase maintenance_work_mem
Temporarily increasing the maintenance_work_mem configuration variable when loading large amounts of data can lead to improved performance. This will help to speed up CREATE INDEX commands and ALTER TABLE ADD FOREIGN KEY commands. It won't do much for COPY itself, so this advice is only useful when you are using one or both of the above techniques.
14.4.6. Increase checkpoint_segments
Temporarily increasing the checkpoint_segments configuration variable can also make large data loads faster. This is because loading a large amount of data into PostgreSQL will cause checkpoints to occur more often than the normal checkpoint frequency (specified by the checkpoint_timeout configuration variable). Whenever a checkpoint occurs, all dirty pages must be flushed to disk. By increasing checkpoint_segments temporarily during bulk data loads, the number of checkpoints that are required can be reduced.
Postico 1 3 3 – A Modern Postgresql Client Documentation Download
14.4.7. Disable WAL Archival and Streaming Replication
When loading large amounts of data into an installation that uses WAL archiving or streaming replication, it might be faster to take a new base backup after the load has completed than to process a large amount of incremental WAL data. To prevent incremental WAL logging while loading, disable archiving and streaming replication, by setting wal_level to minimal, archive_mode to off, and max_wal_senders to zero. But note that changing these settings requires a server restart.
Aside from avoiding the time for the archiver or WAL sender to process the WAL data, doing this will actually make certain commands faster, because they are designed not to write WAL at all if wal_level is minimal. (They can guarantee crash safety more cheaply by doing an fsync
at the end than by writing WAL.) This applies to the following commands:
CREATE TABLE AS SELECT
CREATE INDEX (and variants such as ALTER TABLE ADD PRIMARY KEY)
ALTER TABLE SET TABLESPACE
CLUSTER
COPY FROM, when the target table has been created or truncated earlier in the same transaction
14.4.8. Run ANALYZE Afterwards
Whenever you have significantly altered the distribution of data within a table, running ANALYZE is strongly recommended. This includes bulk loading large amounts of data into the table. Running ANALYZE (or VACUUM ANALYZE) ensures that the planner has up-to-date statistics about the table. With no statistics or obsolete statistics, the planner might make poor decisions during query planning, leading to poor performance on any tables with inaccurate or nonexistent statistics. Note that if the autovacuum daemon is enabled, it might run ANALYZE automatically; see Section 23.1.3 and Section 23.1.6 for more information.
14.4.9. Some Notes About pg_dump
Postico 1 3 3 – A Modern Postgresql Client Documentation Requirements
Dump scripts generated by pg_dump automatically apply several, but not all, of the above guidelines. To reload a pg_dump dump as quickly as possible, you need to do a few extra things manually. (Note that these points apply while restoring a dump, not while creating it. The same points apply whether loading a text dump with psql or using pg_restore to load from a pg_dump archive file.)
By default, pg_dump uses COPY, and when it is generating a complete schema-and-data dump, it is careful to load data before creating indexes and foreign keys. So in this case several guidelines are handled automatically. What is left for you to do is to:
Postico 1 3 3 – A Modern Postgresql Client Documentation Example
Set appropriate (i.e., larger than normal) values for maintenance_work_mem and checkpoint_segments.
If using WAL archiving or streaming replication, consider disabling them during the restore. To do that, set archive_mode to off, wal_level to minimal, and max_wal_senders to zero before loading the dump. Afterwards, set them back to the right values and take a fresh base backup.
Experiment with the parallel dump and restore modes of both pg_dump and pg_restore and find the optimal number of concurrent jobs to use. Dumping and restoring in parallel by means of the -j option should give you a significantly higher performance over the serial mode.
Consider whether the whole dump should be restored as a single transaction. To do that, pass the -1 or --single-transaction command-line option to psql or pg_restore. When using this mode, even the smallest of errors will rollback the entire restore, possibly discarding many hours of processing. Depending on how interrelated the data is, that might seem preferable to manual cleanup, or not. COPY commands will run fastest if you use a single transaction and have WAL archiving turned off.
If multiple CPUs are available in the database server, consider using pg_restore's --jobs option. This allows concurrent data loading and index creation.
Run ANALYZE afterwards.
At bother increasing maintenance_work_mem; rather, you'd do that while manually recreating indexes and foreign keys afterwards. And don't forget to ANALYZE when you're done; see Section 23.1.3 and Section 23.1.6 for more information.